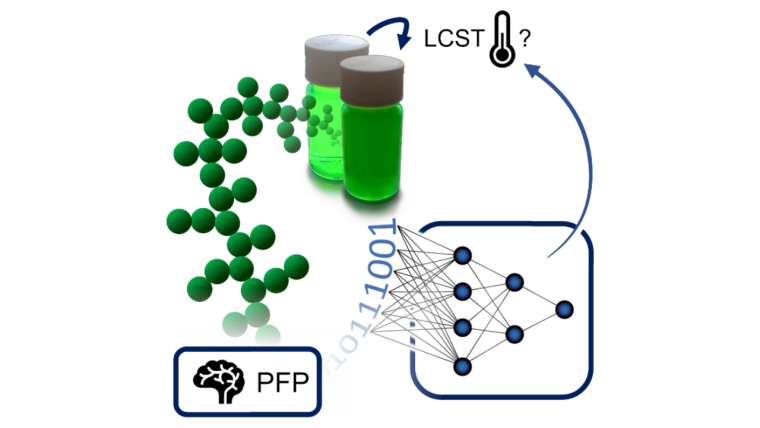
Published:
With increasing awareness of resource scarcity, it is important to develop a resource-efficient path for research in materials science. Modern algorithms based on machine learning make it possible to establish efficient experiment planning so that experiments can be carried out in a targeted manner. However, such algorithms have so far only rarely been applied in polymer research, in particular since the chemical structure of the polymers has so far been difficult to transfer into machine-readable formats.
A new study takes up this challenge and develops a new "fingerprint" for polymers and their chemical structure so that they can be read directly by a computer. In this way, modern machine learning algorithms can be utilized more easily and in a more targeted manner. This new approach could be applied to predict the lower critical solution temperature (LCST) of polymers revealing a very good agreement between experimental results and the values predicted by the machine learning algorithm.
The complete article can be found here: https://www.sciencedirect.com/science/article/pii/S2666386423003508?via%3DihubExternal link
Authors: Yannik Köster, Julian Kimmig, Dr. Stefan Zechel, Prof. Dr. Ulrich S. Schubert